What are Diffusion Models?
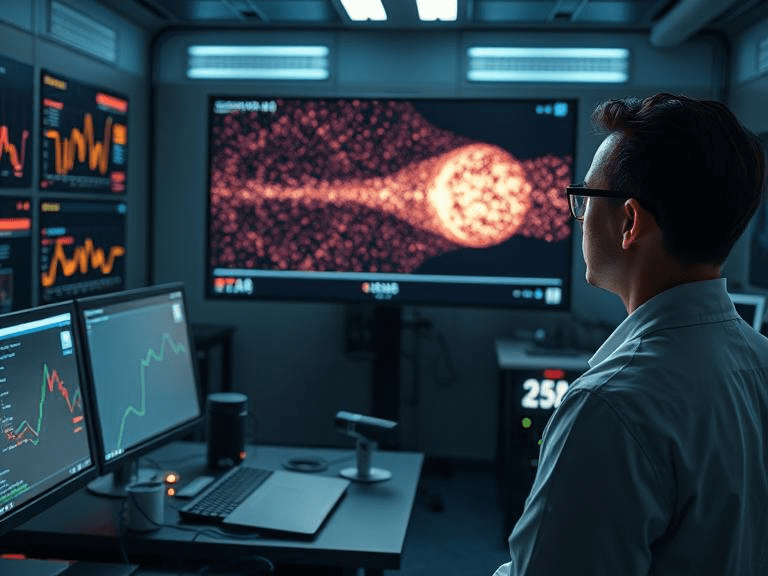
Diffusion model, to understand diffusion model, one must have a sound understanding about the basics of artificial intelligence. As such these models fall under the criteria of a generative artificial intelligence.
To understand the diffusion model in detail, let us first discuss what a diffusion model is and how it works.
What are diffusion models?
Diffusion model works on the principle of generative artificial intelligence thus it is able to generate and manipulate the raw data by processing them as per the training data that was provided to them initially.
For instance, a diffusion model can generate a realistic image just from the noise. To attain more knowledge about the same, one should learn about the generative models such as generative adversarial networks as such they paint a bigger and more complex picture to the future of the technology.
That being said, it is safe to say that the diffusion model is a dynamic part of generative artificial intelligence and machine learning. With the help of machine learning and artificial intelligence, diffusion models can simulate a large number of functions with ease while delivering a high rate of precision and accuracy.
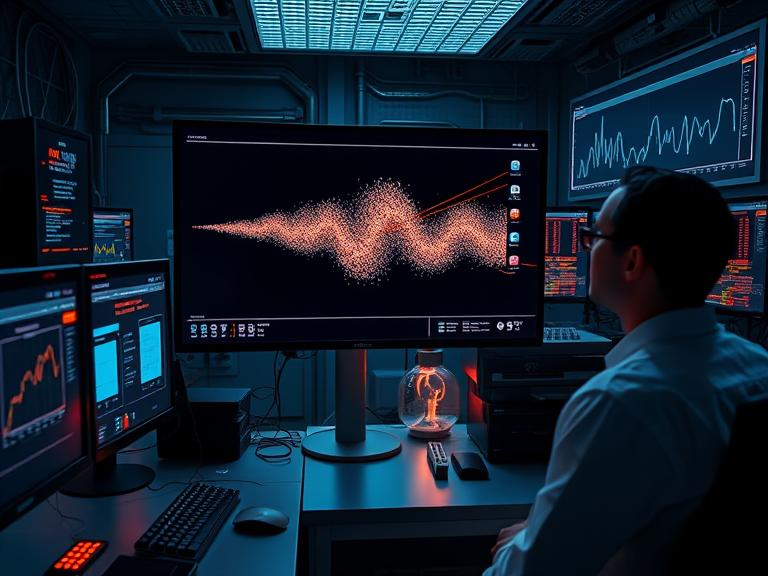
Now let us understand some of the key factors of the diffusion models, which are mentioned below.
Working principle of diffusion model:
As we have established that these are generative models, that means this gain an understanding of reversing the diffusion process in order to produce the required data. Due to this step, the initial noise gets transformed into pure noise, which results in the transformation of simple distribution into a more complex distribution data.
Normally, the models work on a reverse diffusion concept where the data is allowed to interact in a forward manner and then extract the new data in the reverse manner. By following the same trend or pattern, these start their process through the noise and then slowly move towards denoising it to attain data as per requirement.
Key fundamentals of diffusion model:
Diffusion models are categorized into three major parts, and they are mentioned below:
1. Forward diffusion method: In forward diffusion, noise is allowed to interact with the data gradually until it reaches the desired place. For instance, the data is broken down into several small steps, and with passing of each step, the noise is introduced, making the data more random until the data becomes pure noise.
2. Reverse diffusion method: Reverse diffusion works in a just opposite way as compared to the forward diffusion process. As such in this process, it starts from the pure noise and then gradually removes the noise in order to match the data as per the training distribution.
3. Score function: This function helps the reverse diffusion method to estimate the gradient of the data distribution regarding the noise to produce more realistic samples.
Areas where diffusion models are used:
Diffusion models are used in sectors where there is a need to generate a sample or entirely new data from the old one. This generation of new data is very helpful in day-to-day life. That being said, let us discuss some of the well-known applications of these that are used more than one might think. They are as follows:
1. Speech synthesis: Through this function, a diffusion model can create a human-like speech through various audio signal distributions.
2. Image generation: Diffusion models can be used to create real and high quality images from the noise provided. This helps the user to build a dataset to train various other machine learning models.
3. Anomaly detection: Diffusion model can find out any anomalies by comparing the current data distribution changes with the normal data distribution.
4. Data augmentation: This application is used to enhance the performance of the machine learning model by augmenting the current dataset with the new synthetic samples for better results.
Limiting factors of Diffusion models:
1. Slow sampling: As such, it runs on the reverse diffusion concept therefore it needs to satisfy many stages, making its sampling speed slow.
2. Memory usage: Diffusion models consumption of memory is quite high because of the requirement to store various steps while training.
3. Computationally intensive: Due to various complex steps, computational resources are required.
4. Complexity: The Diffusion model is tedious to implement due to its complex architecture and training procedure.
5. Resource demand: It cannot be used in small-scale research centers or applications with restricted resources; as such, it needs high-performing GPUs and TPUs.
6. Fine-tuning: To achieve optimal performance, diffusion models need to carefully tune noise schedules and hyperparameters.
Advantages and disadvantages of diffusion model usage:
Advantages of diffusion model:
● Strategic insights: With the help of strategic insights, the users can adapt and make a well informed decision about their product as such it can change the projection of their product from a failed product to an extremely successful product.
● Novel image techniques can help the user to create a completely new output from the existing input.
● Behaviour understanding: This technique is used to decode the behaviour of human beings for user’s and find out why they make certain behaviour decisions in order to sell their products.
Disadvantages of the diffusion model:
● Training data privacy issue: Due to the large number of data to be processed, there are scenarios where there is a possible chance of leak in the system.
● Complex prompt difficulties: Spatial and numerical components input makes the process of diffusion model little difficult.
● Limited scope
Conclusion:
Diffusion model is a sub part of artificial intelligence because of that It is well established that the diffusion model is based on the generative model of artificial intelligence. It provides very developed data through a stable process, making it ideal for the usage of various applications which we have mentioned above.
Speech analysis, image generation, data augmentation, and anomaly detection are some of the key sectors that use diffusion models. It is safe to say that there is plenty of room for development, and as time passes, there will be more advancement and will be accessible for small-scale research centers also on contrary to the present day status.